Invited Talks
Luís A. Alexandre "Improving Reinforcement Learning by Learning from Demonstrations"
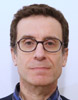
http://www.di.ubi.pt/~lfbaa/
One of the main approaches to learning, that is used in hard cases where it is unfeasible to approach the problem using a supervised training paradigm, is reinforcement learning (RL). It is typically used to learn policies, that map states to actions, for robotics. One issue with RL is the need for long learning sessions, sometimes millions of trials, until a proper policy is obtained. This is time consuming and can usually also be risky since while a robot is learning how to perform a new task through RL, it might attempt to perform actions that can endanger it or its surroundings.
Learning from demonstrations (LfD) is a learning paradigm where a model learns by looking at a demonstration of the task. This makes it much simpler to teach a robot a new behavior and can be used to solve the above mentioned issue of RL safety. Nonetheless, LfD also has issues, like the difficulty for some approaches to learn long time horizon tasks.
In this talk we will present some recent results that show how LfD can be used to both learn tasks with longer episodes and/or sparse rewards and also help RL methods work in a safer way.
Aleksander Byrski "Authorized metaheuristics in action"
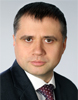
https://home.agh.edu.pl/~olekb/
Aleksander Byrski (Ph.D. 2007, D.Sc. 2013 - AGH University of Science and Technology, Full Professor Title - President of Poland) works at the AGH University of Science and Technology in Krakow, the biggest Technical University in Poland. He is interested mainly in metaheuristic-based optimization, in particular novel techniques related to agent-oriented inspirations, efficient and efficacious implementation of metaheuristics, and novel ideas for metaheuristics inspirations. He holds the position of Deputy Director of the Institute of Computer Science AGH and V-ce Chairman of the Polish Academy of Sciences Computer Science Committee.
Aleksander Byrski (Ph.D. 2007, D.Sc. 2013 - AGH University of Science and Technology, Full Professor Title - President of Poland) works at the AGH University of Science and Technology in Krakow, the biggest Technical University in Poland. He is interested mainly in metaheuristic-based optimization, in particular novel techniques related to agent-oriented inspirations, efficient and efficacious implementation of metaheuristics, and novel ideas for metaheuristics inspirations. He holds the position of Deputy Director of the Institute of Computer Science AGH and V-ce Chairman of the Polish Academy of Sciences Computer Science Committee.
Włodzisław Duch "Understanding real brain neurodynamics using recurrence analysis"
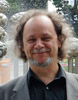
Link to CV: http://www.is.umk.pl/~duch/cv/cv.html
Brain networks may be modeled using attractor neural networks. Dynamics of such networks is characterized by metastable states. Recurrence analysis is very well suited to find such states in neuroimaging or in electrophysiological EEG or MEG signals. Interpretation of brain signals has high diagnostic value, opening also new doors to therapeutic interventions. Unfortunately such signals are nonlinear, noisy, oscillatory, and nonstationary, therefore very difficult to interpret. One promising approach is based on Recurrence Quantification Analysis (RQA) that provides useful features for classification of EEG and other brain signals. RQA measures have been better suited for the quasi-periodic dynamics and require some adjustment in application to the attractor dynamics. To characterize global states of the brain signals in short time windows are represented by power spectra. We have recently showed that this method generates useful RQA features, and that using these features in combination with SVM analysis we can find reduced number of electrodes/features that lead to a high accuracy classification of these complex signals. Identification of recurrent states and analysis of transition sequences between these states provides important information about specific, frequently occurring states, including their spatial locations and dominating oscillation frequency. Connections between observed states and probability of transitions between them can be displayed in graphical form, and linked to the sources in the brain that generate signals measured on the scalp. Some examples of the usefulness of such analysis in diagnostics of mental disorders will be provided. We are developing a BrainPulse software package to automatize such analysis.
Furman Ł, Tołpa K, Minati L, Duch W. (2022) Short-Time Fourier Transform and Embedding Method for Recurrence Quantification Analysis of EEG Time Series.
European Physical Journal Special Topics, 1-15
Janusz Kacprzyk "Small data but big problems: from context-aware to intention-aware flexible bipolar querying"
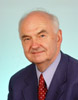
Full member, Polish Academy of Sciences
Member, Academia Europaea
Member, European Academy of Sciences and Arts
Member, European Academy of Sciences
Member, International Academy for Systems and Cybernetic Sciences (IASCYS)
Foreign member, Bulgarian Academy of Sciences
Foreign member, Finnish Society of Sciences and Letters
Foreign member, Royal Flemish Academy of Belgium for Sciences and the Arts (KVAB)
Foreign member, Spanish Royal Academy of Economic and Financial Sciences (RACEF)
Systems Research Institute, Polish Academy of Sciences
Ul. Newelska 6, 01-447 Warsaw, Poland
Email: kacprzyk@ibspan.waw.pl
Google: kacprzyk
Janusz Kacprzyk is Professor of Computer Science at the Systems Research Institute, Polish Academy of Sciences, WIT – Warsaw School of Information Technology, AGH University of Science and Technology in Cracow, and Professor of Automatic Control at PIAP – Industrial Institute of Automation and Measurements in Warsaw, Poland. He is Honorary Foreign Professor at the Department of Mathematics, Yli Normal University, Xinjiang, China. He is Full Member of the Polish Academy of Sciences, Member of Academia Europaea, European Academy of Sciences and Arts, European Academy of Sciences, International Academy of Systems and Cybernetics (IASCYS), Foreign Member of the: Bulgarian Academy of Sciences, Spanish Royal Academy of Economic and Financial Sciences (RACEF), Finnish Society of Sciences and Letters, Flemish Royal Academy of Belgium of Sciences and the Arts (KVAB), National Academy of Sciences of Ukraine and Lithuanian Academy of Sciences. He was awarded with 8 honorary doctorates. He is Fellow of IEEE, IET, IFSA, EurAI, IFIP, AAIA, I2CICC, and SMIA. His main research interests include the use of modern computation computational and artificial intelligence tools, notably fuzzy logic, in systems science, decision making, optimization, control, data analysis and data mining, with applications in mobile robotics, systems modeling, ICT etc. He authored 7 books, (co)edited more than 150 volumes, (co)authored more than 650 papers, including ca. 150 in journals indexed by the WoS. He is listed in 2020 and 2021 ”World’s 2% Top Scientists” by Stanford University, Elsevier (Scopus) and ScieTech Strategies and published in PLOS Biology Journal. He is the editor in chief of 8 book series at Springer, and of 2 journals, and is on the editorial boards of ca. 40 journals.. He is President of the Polish Operational and Systems Research Society and Past President of International Fuzzy Systems Association
Though big data is like mantra in recent times, one should bear in mind that an overwhelming majority of human activities, also in business, proceeds on a small scale and concerns rather “small data” than big data. Unfortunately, this does not always make things easier.
A good example, which will be used for illustration, is an example of a real estate agency that sells apartments (and houses), and serves the customers in an interactive way, that is, by finding apartments that best suit the customers’ intentions and preferences and which are available in a database of the real estate agent that is of course „small”, or in some other „small” databases the agent has access to. Clearly, all these databases are far from what is meant as „big data”.
The main problem is first to retrieve from the customer what he/she is really looking for in the sense of what apartment the customer is interested in, and also to determine preferences among candidate apartments, both on the basis of „internal” characteristics, that is, which apartment characteristics are welcome by the particular customer, and some „external” characteristics, that is, what is available in the particular geographic location or region, in current market situation, etc.
We are concerned first with some approaches to flexible database querying proposed by us (Kacprzyk and Zadrożny) which include queries with (fuzzy) linguistic quantifiers that can aggregate the satisfaction of, e.g., most, almost all, etc. search criteria. For instance, if the criteria are „affordable” and „well located”, then they can be represented by the satisfaction of „most out of {low price, good loan conditions, etc.}” and „most out of {close to park, close to public transportation, safe, etc.}” for „affordable” and „well located”, respectively. Generally, „affordable” and „well located” can be derived by using some ontologies and human-computer interface.
Then, we present - as a next step towards a better reflection of the human intentions and preferences – our concept of a bipolar query the essence of which is that an apartment is sought which is, for instance, „affordable” and, if possible, „well located”. This is meant as that the first condition, „affordable”, is a necessary condition which should be satisfied while the second condition, „well located”, is an optional condition the satisfaction of which is just if possible, not necessary. That is, „and if possible” is not equivalent to the connection „and” and is represented by other means exemplified by some more sophisticated logical expressions. The bipolar queries can then be extended into compound or hierarchical queries in which a synergistic combination of bipolar queries and queries with linguistic quantifiers is involved. This all can help better represent the customer’s intentions and preferences.
Then, to provide an extension from the account for local data, that is, included in the real estate agent’s database, to an additional account for external data, related to the environment, we present new bipolar queries with context. This is to express the meaning of query conditions, for instance, „affordable” and „well located”, to what is valid ”here and now”, that is, what is meant to be affordable and well located in a particular part of the city and currently. Moreover, it is also shown the use of some „global” aspects exemplified by taking into account data on prices in similar cities and changing patterns of what „well located” means, for instance, because of a growing popularity of physical exercise, bicycle riding, etc. This is clearly a passive account for context.
We also show our approach to an active use of context via the so-called context seeking which boils down to finding contexts, e.g. in which parts of the city, in which price ranges, etc. some individual apartments offered by the particular real estate agent stand out from among the available ones. This can provide a further suggestion to the customer and is an example of an active use of context.
The two above uses of context are shown in the perspective of the so-called context awareness which is a well-known characteristic of many systems, notably various intelligent systems like those used in autonomous driving.
This context aware setting is extended to a qualitatively new setting, the so-called intention awareness, the essence of which is the reduction and facilitation of the interaction between the computer system and its user, here the real estate agent and the customer, by trying to predict which action the user will take next. Some possible solutions will be surveyed, exemplified by a Bayesian network based approach, and also some other more specific approaches exemplified by the use of pattern detecting tools based on neural networks, decision trees, etc. A proposal for extending the context aware bipolar queries into the intention aware ones is shown. An example related to an interaction between the real estate agent and customer is provided.
Artur Luczak "Predictive neurons: bio-inspired ideas to improve AI"
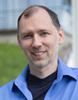
Dr. Luczak is a professor at the Canadian Centre for Behavioural Neuroscience at the University of Lethbridge. He received PhD from the Jagiellonian University, and later he also worked at Yale, Rutgers, and Stanford University. His laboratory uses experimental and computational methods to study how the brain learns and processes information, and how this is distorted in neurological disorders such as epilepsy. For his research contributions, Dr. Luczak was elected to the College of the Royal Society of Canada.
Understanding how the brain learns may help us to build machines with human-like intellectual capacities. Recently, we showed that the ability of a single neuron to predict its future activity may be a crucial element of the learning mechanism in the brain. We tested this predictive neuron idea in neural network simulations and in data recorded from awake animals. This suggests that to achieve AGI, artificial neurons may need to implement predictions and thus be more complex.
Luczak A, McNaughton BL, Kubo Y. Neurons learn by predicting future activity. Nature Machine Intelligence, 2022 https://www.nature.com/articles/s42256-021-00430-y
Zbigniew Michalewicz "On the Significance of Dragon Years in a Life of a Scientist"
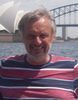
Zbigniew Michalewicz received the Master of Science degree from the Technical University of Warsaw, Warsaw, Poland, in 1974; the Ph.D. degree from the Institute of Computer Science, Polish Academy of Sciences, Warsaw, in 1981, and the D.Sc. degree in Computer Science from the Polish Academy of Science in 1997. He is currently Emeritus Professor of Computer Science at the University of Adelaide, Australia. He is also a Professor with the Institute of Computer Science, Polish Academy of Sciences, the Polish-Japanese Institute of Information Technology, Warsaw, and the State Key Laboratory of Software Engineering, Wuhan University, Wuhan, China. He is also associated with the Structural Complexity Laboratory, Seoul National University, South Korea. Zbigniew Michalewicz is the Chief Scientific Officer at Complexica (www.complexica.com), a leading provider of software applications that harness the power of Artificial Intelligence and big data to improve the effectiveness of sales & marketing activities. For many years his research interests were in the field of evolutionary computation. He published several books, including a monograph Genetic Algorithms + Data Structures = Evolution Programs (3 editions, a few translations, over 20,000 citations, source: Google Scholar), and over 300 technical papers in journals and conference proceedings that are cited widely (50,000 citations, source: Google Scholar). Other books include Adaptive Business Intelligence and How to Solve It: Modern Heuristics (both published by Springer, Berlin, 2006 and 2004, respectively), Puzzle-based Learning (Hybrid Publishers, Melbourne, 2008), Winning Credibility: A Guide for Building a Business from Rags to Riches (Hybrid Publishers, Melbourne, 2007), where he described his business experiences over the last years.
Zbigniew Michalewicz was one of the editors-in-chief of the Handbook of Evolutionary Computation and the general chairman of the First IEEE International Conference on Evolutionary Computation held in Orlando, June 1994. In December 2013 Zbigniew was awarded (by the President of Poland, Mr. Bronislaw Komorowski) the Order of the Rebirth of Polish Polonia Restituta – the second highest Polish state decoration civilian for outstanding achievements in the field of education, science, sports, culture, arts, economy, national defence, social activities, the civil service and the development of good relations with other countries.
The talk presents a personal account of many years’ adventure with science, from early discovery of puzzles, through research in Evolutionary Computation, to working full-time in business – successfully implementing AI – based algorithms for some of the world’s largest corporations. A few applications are briefly discussed that transform data into decisions – these applications are based on work done for three companies (NuTech Solutions, SolveIT Software, and Complexica) over the last 24 years. The talk concludes with some observations and insights. The presentation aims at graduate students and young researchers, who are just about to embark on their professional journey in academia or industry.
Witold Pedrycz "Data Privacy, Energy Awareness, Credibility and Interpretability of Models: Challenges in Machine Learning"
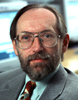
and
Systems Research Institute, Polish Academy of Sciences, Warsaw, Poland
We have been witnessing spectacular achievements of Machine Learning (ML) with highly visible accomplishments encountered, in particular, in natural language processing and computer vision impacting numerous areas of human endeavours. Driven inherently by the technologically advanced learning and architectural developments, ML is highly impactful coming with far reaching consequences; just to mention autonomous vehicles, control, health care imaging, decision-making in critical areas, among others.
We advocate that the design and analysis of ML constructs have to be carried out in a holistic manner by identifying and addressing a series of central and unavoidable quests coming from industrial environments and implied by a plethora of requirements of interpretability, energy awareness (being also lucidly identified on the agenda of green AI), efficient quantification of quality of ML constructs, their brittleness and conceptual stability coming hand in hand with the varying levels of abstraction. They are highly intertwined and exhibit relationships with the technological end of ML. As such, they deserve prudent attention, in particular when a multicriterial facet of the problem is considered.
The talk elaborates on the above challenges and identifies the linkages among them. In the pursuit of coping with such quests, we advocate that Granular Computing can play a pivotal role offering a conceptual environment and realizing algorithmic development. As a detailed study, we discuss the ideas of knowledge transfer showing how a thoughtful and prudently arranged knowledge reuse to support energy-aware ML computing. We discuss passive and active modes of knowledge transfer. In both modes, the essential role of information granularity is identified. A generalized scenario of multi-source domains is discussed. Relational knowledge distillation leading to model compression and model interpretability is studied.
Jerzy Stefanowski "Using prototypes and counterfactuals in explaining machine learning models: selected methods and research challenges"
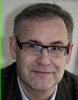
Poznań University of Technology, Poznań, Poland
Jerzy Stefanowski graduated from the Faculty of Electrical Engineering of the Poznań University of Technology in Poland in 1987. Since that year he has been employed at the Institute of Computer Science this university and received subsequent scientific degrees, Ph.D with honors in 1994 and the degree of habilitated doctor (D.Sc). In December 2021, he was elected a corresponding member of the Polish Academy of Sciences.
Since Dec. 2022 he heads the new Machine Learning lab at Poznan University of Technology (which he founded), previously he was also a head of the other lab of intelligent decision support systems at the same university.
His research interests include data mining, machine learning and intelligent decision support. Major results are concerned with: ensemble classifiers, learning from class-imbalanced data, online learning from evolving data streams, explainable AI, induction of various types of rules, data preprocessing, generalizations of rough set theory, descriptive clustering of texts and medical applications of data mining.
He was a visiting professor or researcher in several universities, mainly in France, Italy, Belgium, Spain and Germany. In addition to his research activities he served in a number of organizational capacities: current vice-president of Polish Artificial Intelligence Society (vice-president since 2014); co-founder and co-leader of Polish Special Interest Group on Machine Learning, the Editor in Chief of Foundations of Computing and Decision Sciences.
More details could be found at http://www.cs.put.poznan.pl/jstefanowski/.
The development of artificial intelligence and machine learning leads to real life applications, which may significantly influence human and society. On the other hand, it exposes the importance of satisfying additional requirements for explaining the automatic decisions of such intelligent systems. Up to now many methods for explaining predictions of black box machine learning models were proposed. In this talk we focus interest on two categories of these explanations: prototypes and counterfactuals. In the first case prediction of the model for the given instance is related to its similarity to selected prototypes - representative examples or their sub-parts from the input training data. We will discuss how this paradigm is implemented in neural prototype networks, deriving from the idea originally presented to explain image classification. Next, we will show this inspiration for analogous solutions for the textual data. In particular we will introduce our proposal of Prototypical Convolutional Neural Network designed for classification of short text documents and sentiment analysis, where prototypes, learned as special embeddings in the last hidden layers, are linked to the most similar phrases in the documents, which are interpretable to human. Results of its experimental evaluation will be also presented. In the other part of this talk we focus our interest on counterfactual explanations. Unlike other explanation methods, they provide recommendations on how to change values of some attributes describing the considered instance in order to get the more desired predictions. Here we present selected methods for tabular data and discuss in more details various measures and approaches to evaluate different explanations generated for the same instance. We will conclude the talk with discussing several open research issues concerning both kinds of these explanations.
Wenying Xu "Fully distributed event-triggered control for multi-agent systems"
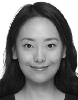
Southeast University, Nanjing 210096, China
Dr. Wenying Xu received M.S. degree in Applied Mathematics from Southeast University, Nanjing, China, in 2014, and the Ph.D. degree from the City University of Hong Kong, Hong Kong, in 2017. She is currently Associate Professor in the School of Mathematics, Southeast University, China, and is also a Post-Doctoral Fellow in Potsdam Institute for Climate Impact Research, Potsdam, Germany. From 2017 to 2018, she was a Research Fellow in the School of Electrical and Electronic Engineering, Nanyang Technological University, Singapore. She was an Academic Visitor in the Department of Computer Science, Brunel University London, U.K, from May
2015 to Aug. 2015 and from Oct. 2019 to Dec. 2019, and was also a Senior Research Associate in the Department of Mathematics, City University of Hong Kong, Hong Kong, China, from Jun. 2018 to Sep. 2018. Her research interests include cyber-physical system, game theory in networks, distributed event-triggered control, and distributed cooperative control.
Dr. Xu was a recipient of the Outstanding Master Degree Thesis Award from Jiangsu Province, China, in 2015, and an Alexander von Humboldt Fellowship in 2018.
This talk will present recent advances on adaptive dynamic event-triggered (ADET) schemes of multi-agent systems. First, we will discuss the state-based and observer-based consensus control problem of multi-agent systems with general linear dynamics under event-triggered communication (ETC), respectively. Two novel event-triggered strategies named adaptive dynamic event-triggered (ADET) schemes for one-to- all and one-to-one ETC are well developed, in which on-line triggering parameters associated with each node or edge and dynamic thresholds with updating laws are introduced, respectively. Second, the hybrid Nash equilibrium (NE) seeking problem over a network in a partial-decision information scenario.
In order to save communication cost, a novel event-triggered scheme, namely, edge-based adaptive dynamic event-triggered (E-ADET) scheme, is proposed with on-line-tuned triggering parameter and threshold, and such a scheme is proven to be fully distributed and free of Zeno behavior. Then, a hybrid NE seeking algorithm, which is also fully distributed, is constructed under the E-ADET scheme. By means of the Lipschitz continuity and the strong monotonicity of the pseudo-gradient mapping, we show the convergence of the proposed algorithms to the NE. Compared with the existing distributed algorithms, our algorithms remove the requirement on global information, thereby exhibiting the merits of both flexibility and scalability.
Shaofu Yang "Distributed Optimization via Coordination in Multi-Agent Systems"
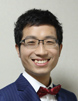
Southeast University, Nanjing 210096, China
Dr. Shaofu Yang received the Ph.D. degree in engineering from the Department of Mechanical and Automation Engineering, Chinese University of Hong Kong, Hong Kong, in 2016. He was a Postdoctoral Fellow with the City University of Hong Kong, Hong Kong, in 2017. He is currently an Associate Professor with the School of Computer Science and Engineering, Southeast University. His current research interests include distributed optimization, multi-agent systems, and multi-agent learning. He serve as a senior program committee member of ECAI 2023, and a program committee member of ECML-PKDD (2022, 2023), ACML 2021, ICONIP 2021, etc. He was selected into the seventh Youth Talent Promotion Project of China Association for Science and Technology.
Distributed optimization with a sum of objective functions appears widely in a variety of scenarios including sensor networks, formation control, and machine learning. A key challenge for the design of distributed optimization algorithm is how to design the dynamics of an individual agent and local interaction rules among agents. In this report, we will discuss the design of distributed optimization algorithms based on dynamical multi-agent systems, in which the optimal solution is attained when consensus is achieved in a group of collaborative agents under the situation that each agent knows only a part of the optimization problem. In particular, several continuous-time multi-agent systems for distributed optimization, distributed min-max optimization, and distributed Nash equilibrium seeking will be introduced, which can provide us some insights on the distributed optimization algorithms from the viewpoint of coordination among agents.